Is that a Cat? Teaching Computers how to Learn.
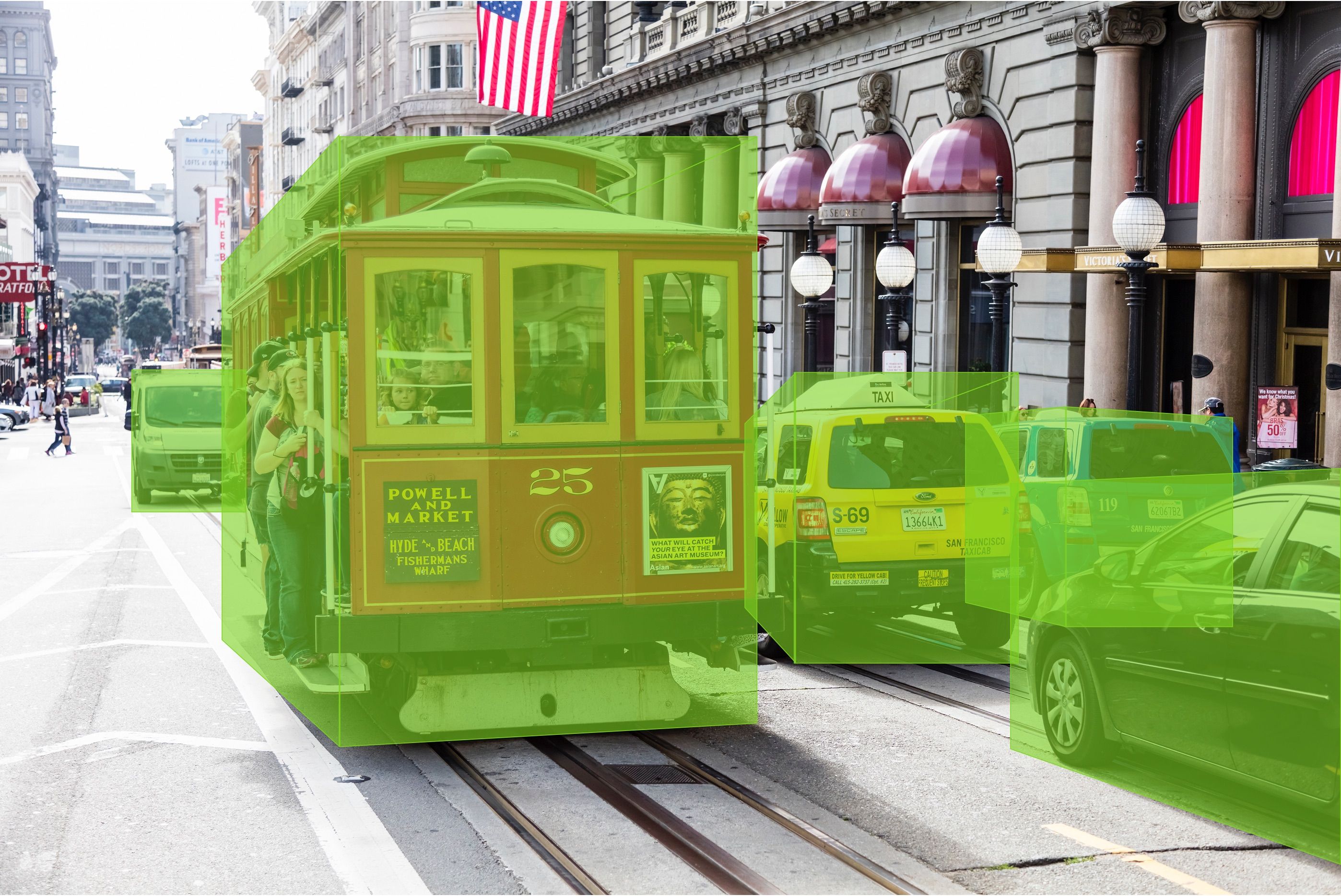
Our partner Mike Volpi shares his thoughts on our latest investment in Scale, a company that aims to accelerates the development of AI by democratizing access to intelligent data.
Artificial Intelligence (AI) and Machine Learning (ML) are two of the hottest topics in tech today. And while arguably the tech community is prone to getting carried away by the latest hype, I view AI and ML as technological themes that will be as impactful as mobile has been for the last decade and the web was for the decade before that. AI/ML will transform so many of the services and applications we use today - and surely for the better. So, I am definitely an AI optimist.
That said, AI is still in it’s infancy. If you compare machine to humans, there are many ways in which humans are still much more efficient and capable. One of them is in vision, perception, and recognition. Thanks to hundreds of thousands of years of evolutionary change, humans have developed an extraordinary capacity to see, recognize and categorize objects quickly. And that is especially impressive given that the human brain operates on less than 20 Watts (consider a high end computer consumes comfortably over 1,000 Watts). The comparison is stark when you think about how quickly and efficiently a baby can learn that the fluffy animal purring around them is a cat versus how long and arduous it is for a computer to learn about cats from images.
Once computers learn how to do certain tasks, they can certainly be extremely efficient. It just takes them a very long time to learn on their own. There are two branches in learning employed in AI. When a computer learns without labeled training data, it is called unsupervised learning. A computer can get to the right answer with unsupervised learning, but it takes them a very long time and it requires *a lot* of data to get there. When a computer learns with labeled training data, it is called supervised learning. The labeled data is the image of a cat with the word “cat” attached to it as metadata. This greatly accelerates the process of learning. These concepts are, in fact, somewhat obvious. A baby can learn a lot faster when a parent tells them it’s a cat versus having to figure it out on their own.
Not surprisingly, the process of creating labeled training data has become a significant and a costly business problem - especially in sectors that can significantly benefit from the application of AI and computer vision. Industries such as self-driving cars, medical diagnoses from imagery, intelligent security cameras, and others work faster and better when AI is trained with label datasets. But where do these labeled datasets come from?
Most of the labels data today are human annotations -- thousands upon thousands of images marked up by humans. This is quite a painfully slow and inefficient process. It involves annotators sitting in front of the computer screen clicking away at imagery, labeling them one by one. And, as the number of images escalates, it’ll only get more expensive and error-prone.
It’s this very problem that our newest Investment -- Scale, and its founder Alex Wang set out to tackle. Alex has always been a precocious entrepreneur. At the ripe old age of 18, he decided to skip college and become an early employee at Quora (shortly after representing the US at the prestigious International Olympiad of Informatics). After a couple of years, he tried his collegiate hand at MIT jumping right into a collection of advanced of ML classes. While earning perfect scores, he didn’t quite find that academia as fulfilling to his entrepreneurial spirit and dropped out to start his own company.
Scale’s initial goal was to scale the process of labeling training data. Interestingly, there are two parts to the problem - one is the task of marshalling thousands of people that label data; and the second is the creation of a sophisticated suite of tools that make those labelers 10 times more efficient and accurate. Alex and his team took on these challenges two years ago and have been blazing a trail ever since. In the process, they’ve assembled a world-class technical team of engineers and technologists that are transforming the space.
For a company this young, Scale has accumulated a remarkable number of high-profile customers particularly in the autonomous driving, healthcare and consumer goods sectors. All of these companies need large amounts of accurately labeled data at very low costs, which make Scale’s service an attractive proposition. In the self-driving category, Scale has become the sine qua non of labeled data, with industry leaders such as Lyft, Zoox, and others relying on them to obtain accurate training data.
As successful as the company has been so far, they’ve only just started to scratch the surface. Given the increasing number of applications that will use computer vision, Scale’s opportunity is simply massive. We believe that they have the potential to become the AWS of the AI world.
Today, we’re delighted to announce our investment in Scale. At Index, we’re betting big on AI, but what really gets us excited is backing entrepreneurs that we admire - and Alex Wang is certainly a special one. With AI in its early innings, we’ve no doubt that Alex and his team will play a big part in its evolution and we look forward to joining along for the ride.
Published — Aug. 7, 2018