Compute is king: Our investment in Phaidra
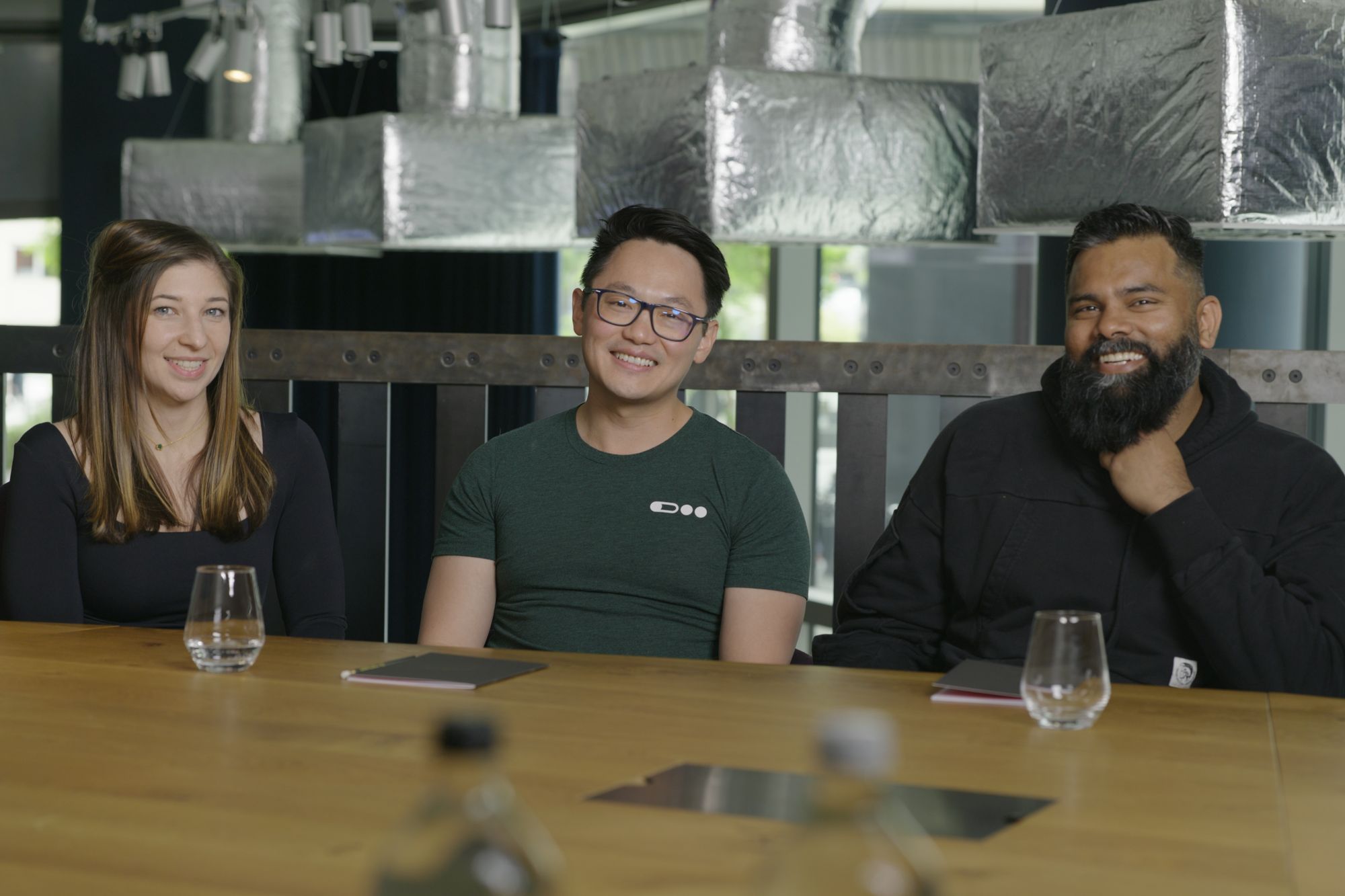
Compute is king. It always has been—just look at NVIDIA’s market cap. But the rapid increase in high-powered GPUs, driven by advancements in AI, is creating not only growing demand for compute but also a looming energy crisis that few are talking about.
In most technologies, scaling compute is logarithmic. You might see immense gains initially, but they diminish over time. In SaaS systems, overprovisioning a cluster doesn’t improve the customer experience beyond a certain point. In AI land, however, things are different. Scaling is exponential. When you compute 10x, you get 100x the performance of the model and customer experience (roughly speaking). This explains the rush to buy GPUs by AI companies and the subsequent rapid growth in compute infrastructure. From Sam Altman purportedly seeking up to $7 trillion to build chip factories, to hyperscalers buying up rural land for data centers, to NVIDIA’s soaring earnings, the rising demand for compute is a problem that will only accelerate as AI technologies advance.
"Scaling compute capacity is critical for AI innovation, but it comes with a significant cost: energy. Phaidra is solving this core power problem and unblocking the industry to increase compute infrastructure worldwide."
But deploying increased compute infrastructure creates another huge and largely overlooked problem: how does one power and cool these chips? We’ll spare you the gnarly details of increasing wattage—but suffice it to say, the need for power scales significantly with each leap in compute capacity. This escalating power usage is intricately linked to cooling, as cooling systems themselves consume considerable energy to manage the heat generated by more powerful servers. And while powering the servers is relatively straightforward, the most significant efficiency gains come from optimizing cooling systems, which can greatly reduce overall energy use. This is critical at a time when certain regions are so power-constrained that new data center developments are being actively stopped. In Ireland, data centers are projected to account for 32% of national electricity consumption by 2026. Every time we speak to hyperscalers, they lament the rapid increase in power-hungry chips.
Data centers have always been a “dirty” business, but the increasing power consumption demanded by these chips, combined with the rapid scaling of compute needed to enable generative AI, is the burning problem standing in the way of innovation—and it’s flying under everyone’s radar.
That is, except for the Phaidra team. They’re building an autonomous control system to help data centers and other mission-critical industrial facilities to manage power consumption. The project began in 2016 when Jim Gao worked as a Google data center operator. Inspired by DeepMind’s AlphaGo work, Jim saw the opportunity to curb growing power consumption in Google’s data centers by leveraging machine-learning techniques. His enthusiasm caught the attention of Veda Panneershelvam, a primary research engineer on AlphaGo, and they joined forces at DeepMind Energy. They built and deployed an autonomous AI control agent in Google’s data centers, reducing cooling energy consumption by 40%.
To put that in perspective, cooling can represent 20–40% of the total energy consumed by a data center, so their solution saves millions in annual energy costs. Katie Hoffman, an industrial control systems engineer at Trane Technologies, read about their results and reached out to Jim. She outlined the broader market opportunity, kickstarting a partnership at Google and the eventual spinout of Phaidra.
Led by Jim, Veda, and Katie, Phaidra currently serves multiple large customers in the data center, district energy, and pharmaceutical industries, helping them significantly reduce power consumption. These industrial facilities often use a building management system (BMS) to control components like pumps, chillers, cooling towers, fanwalls, etc. Phaidra’s platform connects as a supervisory layer on top of the BMS, analyzes thousands of sensor trends in real-time, identifies the optimal strategy for operating the cooling system, and sends instructions back to the BMS for automatic implementation.
"Phaidra’s customers have experienced up to 15% energy savings in their first year of deploying the platform, a figure that will automatically increase over time as the AI continues learning."
Phaidra’s AI uses reinforcement learning, a machine learning technique that learns by doing (just like humans). Before the AI even sends its first setpoint change command, it is trained on the historical telemetry data of the specific site it is managing. That way, it understands the nuances of the facility and operates, initially, in a manner similar to the existing operations team. This ensures safe operations. From there, through consistent interactions with the data center cooling system, the AI learns and gets progressively better at reducing power consumption while maintaining stable operations. This type of deep reinforcement learning is complex but massively capable, requiring the extensive expertise of researchers like Veda, who have led work in this field for years. The Phaidra platform allows for seamless integration with existing BMSes, reduces power consumption, and provides visibility into plant operations.
Phaidra’s customers have experienced up to 15% energy savings in their first year of deploying the platform, a figure that will automatically increase over time as the AI continues learning. In addition to energy savings, Phaidra’s customers have found value in two other key areas.
First, the data center industry’s growth is constrained by its ability to find skilled plant operators. Phaidra enables its customers to grow their capacity without requiring as much headcount. Importantly, Phaidra isn’t eliminating jobs but rather automating the mundane, complex, and repetitive tasks historically handled by programmed static controls. The major difference is that Phaidra’s AI can learn, adapt, and improve dynamically as the effectiveness of equipment in the system changes, eliminating the need for costly re-commissioning or re-programming.
Second, Phaidra significantly improves thermal stability—in fact, its AI almost entirely eliminated thermal excursions for a large pharmaceutical customer, leading to improved product quality. While energy savings will continue to be the primary motivator for Phaidra’s customers, we see labor efficiency and improved operational stability as two other key problem areas where Phaidra can deliver immense value.
Scaling compute capacity is critical for AI innovation, but it comes with a significant cost: energy. Phaidra is solving this core power problem and unblocking the industry to increase compute infrastructure worldwide. We’re so excited to partner with the Phaidra team to solve this urgent problem and enable even more scaling to power the future of AI.
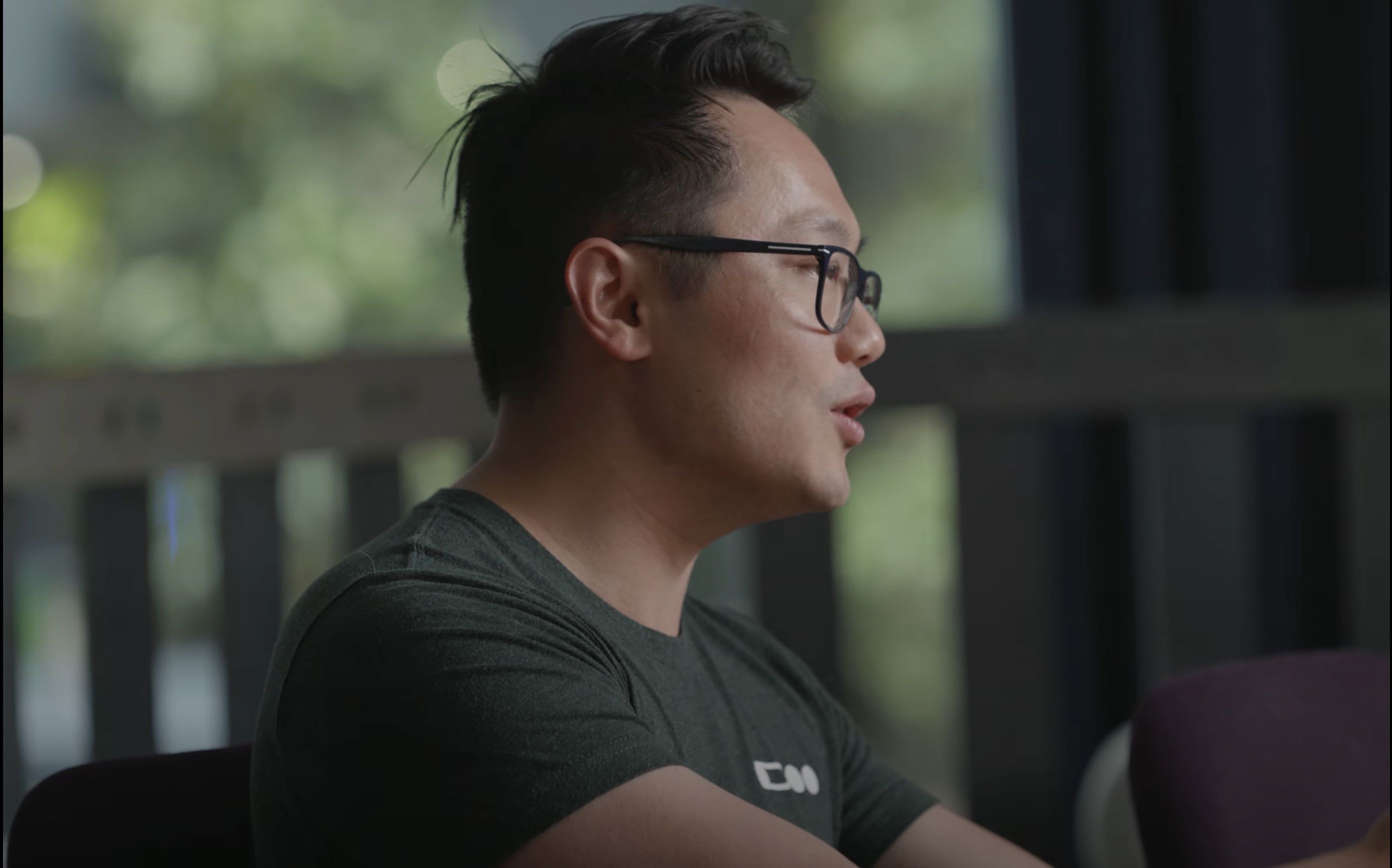
Published — July 2, 2024